In the digital era, one phrase is making waves across industries: Big Data. It’s an intriguing concept, promising to revolutionize the way businesses operate, governments function, and individuals live. But what exactly is Big Data, and why does it matter?
This vast ocean of information we’re swimming in is more than just a collection of facts and figures. It’s a goldmine of insights, waiting to be discovered. Big Data, in essence, is the fuel powering the engine of today’s data-driven world. It’s the key to unlocking a future where informed decisions are the norm, not the exception.
So, let’s dive in and explore this fascinating world of Big Data, its implications, and how it’s reshaping our lives and the world around us.
Understanding Big Data
Unfolding the complexities of Big Data requires first comprehending its fundamental constituents. At the core, it involves vast arrays of data, too colossal for traditional data processing tools to handle. It’s garnered its name, ‘Big Data,’ precisely due to this enormity in volumes, which ranges in petabytes and exabytes.
Unpacking the primary characteristics of Big Data involves examining what’s commonly recognized as the ‘3Vs’ – Volume, Velocity, and Variety. These three elements encapsulate the quintessence of Big Data.
Looking at the ‘Volume’ aspect, it denotes the magnitude of data generated every second. Examples include social media updates like Instagram posts, surveillance footages, and e-commerce transactions. A figure reported by Domo, a cloud-based operating system, offered the startling revelation that humans generate 2.5 quintillion bytes of data every day.
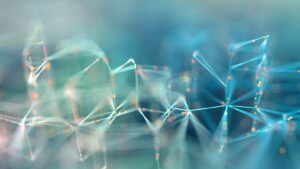
Onward to the ‘Velocity’ aspect, it outlines the rapidity with which data is produced and processed. An enlightening instance is real-time stock exchange data that necessitates instantaneous analysis.
Finally comes ‘Variety,’ and it encapsulates the myriad types of data out there. From structured data like numbers and dates to unstructured data like text, images, and videos, the scope of data variety is indeed quite expansive.
Finally, one must consider several challenges accompany the handling of Big Data. These range from issues of storage and processing to security concerns. Overcoming these obstacles demands innovative solutions, further reinforcing the significance of understanding Big Data in the present scenario.
Key Components of Big Data
Emerging from the ‘3Vs’ concept, Big Data encompasses additional components, contributing directly to its vast potential and accompanying challenges. Three pivotal components making up a comprehensive Big Data strategy, include data sources, data storage and data processing.
Data Sources
Data sources, the raw material for Big Data, occupy an essential place in the strategy. These are, essentially, origins where data comes from. These could be diverse, ranging from business transactions, social media interactions, machine-generated logs, and even sensors in Internet of Things (IoT) devices. Each source, such as a customer transaction record, contributes a tiny bit to the data ecosystem, yet when viewed as a whole, plays a vital part in the broader Big Data narrative.
Data Storage
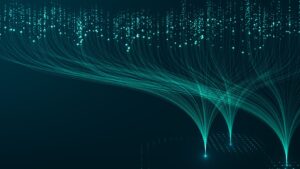
Next in line is data storage, a crucial challenge for Big Data given the massive volume involved. Companies rely on a variety of options, each with its specific benefits and drawbacks. Traditional databases, for example, provide consistency and simplicity, yet often struggle with capacity constraints. In contrast, newer technologies like Hadoop Distributed File System (HDFS) and Google Cloud Storage offer enhanced scalability, capable of accommodating vast volumes of data.
Data Processing
Lastly, data processing stands as the third pillar, transmuting raw data into actionable insights. This step often involves data analytics tools, machine-learning algorithms, and querying languages. For instance, Spark and Flink, both popular processing frameworks, allow for complex calculations and real-time data processing. As a consequence, data processing stands as the backbone of data-driven decision making, underpinning the potential of Big Data in delivering crucial industry insights.
Big Data Technologies and Tools
Unearthing valuable insights from big data necessitates the usage of powerful technologies and tools. From data collection to analysis, each stage employs distinct tools that facilitate the effective management of this vast information trove.
Data Collection and Storage Tools
Big Data generation occurs continuously across various data sources, including social media platforms, sensors, and business transactions. Capturing this information requires efficient tools and technologies. Apache Flafka, a robust tool, serves this purpose, pulling in vast amounts of data quickly and reliably. For storing this colossal data, technologies like NoSQL databases and Hadoop Distributed File System (HDFS) are employed. For example, MongoDB, a NoSQL database, is favored for its flexibility, while Hadoop offers a cost-effective solution for storing petabytes of data.
Data Processing Tools
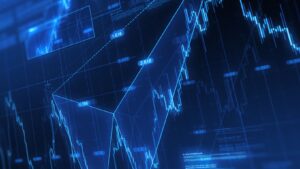
Once data collection and storage are handled, the next challenge is processing this immense volume of data. Apache Hadoop and Spark are key players in this realm, offering robust big data processing capabilities. Hadoop’s MapReduce model enables processing of large data sets across distributed clusters, while Spark’s in-memory computing capabilities make tasks swifter, particularly for data analytics and machine learning applications.
Data Analysis and Visualization Tools
After processing, the data must be analyzed to extract meaningful insights. Tools like Apache Hive and Pig aid in this process by facilitating data quering and analysis on large data sets. Additionally, visualization tools such as Tableau and PowerBI transform complex data sets into easy-to-understand visual representations, enabling stakeholders to quickly comprehend and make informed decisions based on the insights derived.
Pragmatic Applications of Big Data
With a firm understanding of Big Data and its essential tools, the following section details its pragmatic applications. Big Data, far from being an abstract concept, permeates various sectors, delivering valuable insights.
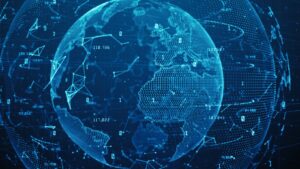
- Healthcare: In healthcare, Big Data offers potential for improved patient care. Medical records, wearable devices and genomics data contribute to the vast pool. Analysis of this data identifies disease trends, improves diagnostics, personalizes treatment, and predicts epidemics.
- Retail and E-commerce: Retailers and E-commerce giants benefit enormously from Big Data. Customer data, from buying habits to social media activity, lays the foundation for personalized marketing campaigns, optimized pricing strategies, and improved inventory management.
- Finance and Banking: For financial institutions, big data helps mitigate risks and ensures regulatory compliance. Transaction data, customer data, and market data feed into fraud detection algorithms, risk modeling, customer segmentation, and algorithmic trading.
- Telecommunications: Telecommunication companies leverage Big Data for network optimization, customer experience management, and predictive maintenance. Real-time data from mobile devices, network equipment, and customer interactions enhance operational efficiency.
- Transportation: Transportation industry uses Big Data for optimized route planning, predictive maintenance, and customer behavior prediction. Traffic data, vehicle data, and passenger data enable smarter, safer, and more efficient operations.
Challenges and Solutions Associated with Big Data
Despite its widespread adoption and transformative potential, Big Data comes with an array of challenges. From data accuracy to security concerns, these obstacles demand sophisticated solutions and strategies.
One significant challenge lies in managing the immense volume of data. Data centers often struggle with storage issues, as traditional storage systems can’t effectively handle the quantity of data generated by sources such as IoT devices and business transactions. An accessible solution to this is the implementation of storage technologies like NoSQL databases and Hadoop Distributed File System (HDFS), both of which offer enhanced scalability.
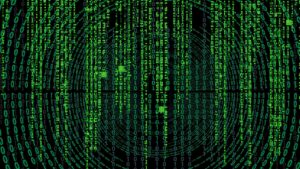
Another challenge surfaces with data velocity. The speed at which data enters networks can overwhelm processing abilities. Fortunately, real-time data processing tools like Apache Flink and Spark can handle high-velocity data flow, allowing for timely insights and decisions.
Data variety presents yet another impediment, given the spectrum of data types and formats in play. Whether structured or unstructured, the diverse data output from various sources poses processing difficulties. Resolving this issue involves the application of tools like Hive and Pig, designed to deal with multiple data formats, thus enabling seamless data analysis and visualization.
While challenges arise in addressing the ‘3Vs’ of Big Data, innovative technologies and tools provide solutions, guiding the rapid progression and potential of Big Data across multiple sectors.
The Future of Big Data
Incorporating insight from the preceding segments, it’s evident that Big Data springs immense potential while posing incalculable challenges. Future trends in Big Data suggest a rise in data generation due to increased IoT devices, burgeoning mobile data usage, and rising business transactions, mandating the necessity for robust data management solutions.
One impelling trend transforms the style of data storage. Traditional databases could give way to newer technologies like hybrid transactional and analytical processing (HTAP) systems. These systems offer the advantage of real-time operational and analytical capabilities, catering to high-volume, high-velocity data.
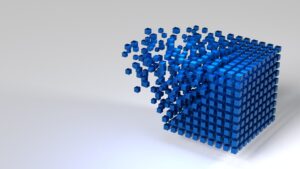
A leap in data processing tools also appears on the horizon. Present-day Hadoop and Spark platforms may evolve to favor adaptive real-time and predictive analytics engines. These advanced tools can handle colossal data streams while offering faster and intuitively perceptive data insights.
Furthermore, advancements in data analytics and visualization tools, such as Apache Hive, Pig, Tableau, and PowerBI, align with the rising demand for simplified yet detailed data interpretation. These tools embody the shift towards data democratization, where even non-tech personnel comprehend data patterns and utilise them for efficacious decision-making.
Data security, a persistent concern associating Big Data, sees a future fortified with stronger encryption algorithms, profound vulnerability assessments, and rigorous data quality checks. An emerging paradigm of privacy-preserving analytics also hints at a future where data privacy is notably prioritized without compromising data utility.
Finally, it appears the future of Big Data rests on adaptability, innovation, and agility. Businesses, institutions, and individuals who adopt and evolve with these prevalent shifts stand poised to leverage the power of Big Data optimally.