In the rapidly evolving digital world, one term that’s creating waves is ‘Machine Learning’. It’s not just a buzzword, but a revolution that’s reshaping our lives and industries. From personalized movie recommendations to accurate weather forecasts, machine learning is the invisible force behind these conveniences.
This technology, often cloaked in complexity, is simpler than you might think. It’s about teaching computers to learn from experience and make decisions based on that. Intrigued? Stay with us as we unravel the world of Machine Learning, its applications, and how it’s paving the way for a smarter future.
The Basics of Machine Learning
Delving deeper into Machine Learning involves understanding its basic concepts. At its core, this technological marvel functions on algorithms. These mathematical models perform specific tasks by taking in input data, processing it, and generating a desired output.
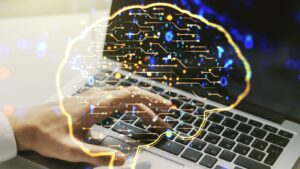
Consider supervised and unsupervised learning, two vital types of Machine Learning. Supervised learning, much like a tutored student, employs labeled dataset for training. The model learns by relating input to output labels. For instance, a weather prediction model would use historical meteorological data, including temperature, humidity, wind speed to predict future weather conditions.
Precisely, Machine Learning brings forth computers that aren’t just programmed, but ones that learn, evolve, and adapt. It’s the key to unlocking even the most sophisticated Artificial Intelligence (AI) systems, poised to revolutionize the world. By understanding these fundamental concepts, one gains a clearer comprehension of this transformative technology.
Types of Machine Learning
In the scope of Machine Learning, there exist three primary types: Supervised Learning, Unsupervised Learning, and Reinforcement Learning. Each type offers unique mechanisms and capabilities.
Supervised Learning
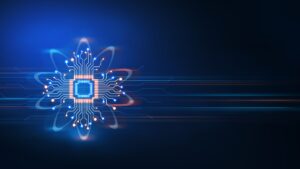
In Supervised Learning, the machine utilizes labeled input data. For instance, an e-commerce site might use Supervised Learning to suggest products to customers. The system gets trained on past purchasing data—calculating the likelihood of a customer buying a product based on their past purchases.
Unsupervised Learning
Unsupervised Learning, on the other hand, detects patterns and relationships in unlabeled data. An example of this approach occurs in social media platforms, where algorithms analyze vast amounts of user data to identify common threads. They can discern groups of people with similar preferences, enhancing targeted advertising practices.
Reinforcement Learning
Different from both Supervised and Unsupervised Learning, Reinforcement Learning operates via a system of rewards and penalties. A classic example here is a chess-playing AI. The AI receives a reward for a successful strategy but suffers a penalty for a losing one. Over time, these rewards and penalties shape the AI’s strategy, improving its performance.
Deep Learning
As an extension of these principles, there’s Deep Learning. Taking cues from human brain functions, this style of Machine Learning focuses on neural networks with multiple hidden layers. Deep Learning models can process vast quantities of data, offering superior predictive capabilities. For instance, they’ve found use in self-driving cars—interpreting road signs and reacting to live traffic to optimize drive routes and ensure passenger safety.
The Importance of Machine Learning in Today’s World
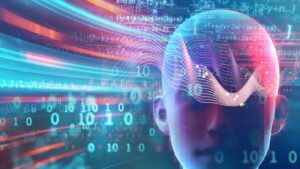
In the current digital age, Machine Learning (ML) holds a pivotal role with its broad spectrum of applications. It’s drastically transforming the way industries function, creating a bridge between humans and machines. For instance, healthcare sectors harness ML for diagnosing diseases, predicting patient outcomes, personalizing treatment, discovering novel drugs, thus, fundamentally reforming patient care. Additionally, in finance, Machine Learning algorithms bolster risk management and fraud detection, notably enhancing security measures.
The paramount importance of Machine Learning in today’s world is unmistakable. It brings about innovative solutions spanning numerous fields, ensuring progressive advancement in technology and pushing the boundaries of what machines can achieve, hence unlocking countless possibilities.
Advantages and Disadvantages of Machine Learning
Machine Learning (ML) offers remarkable advantages, enhancing many aspects of today’s digital world. These include increasing efficiency in process automation, as seen in industries like healthcare for disease diagnosis and personalized treatment. ML also aids in precise prediction making, as demonstrated in the finance sector for risk management. Furthermore, in marketing, ML assists in understanding customer behavior, tailoring strategies for optimized engagement and improved return on investment (ROI).
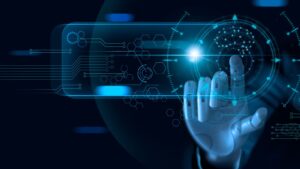
However, the implementation of Machine Learning is not without its disadvantages. One prominent issue is overfitting, wherein the ML model becomes overly complex, excellently performing in training but failing in new, real-world situations. This issue can lead to inaccurate predictions and faulty decision-making.
Despite these drawbacks, it’s clear that the advantages of Machine Learning, ranging from increased process automation efficiency to precise prediction making, generally outweigh the disadvantages. As techniques for managing these issues continue to improve, the revolutionary impact of ML across various fields is set to increase.
Best Practices in Machine Learning
Implementing Machine Learning (ML) in everyday tasks necessitates following best practices. These practices facilitate optimal performance, leading to accurate results and efficiency.
Opt for simplicity. Complex models don’t necessarily guarantee superior results. For instance, linear regression, arguably the simplest ML model, can give excellent results when applied correctly.
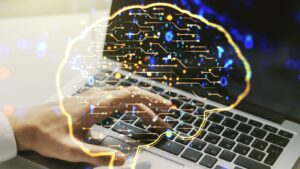
Prioritize data quality over quantity. Investing resources in acquiring more data doesn’t always translate into better performance. Instead, focus on improving data quality by cleaning, preprocessing, and enriching the existing data. The Right Whale Recognition competition on Kaggle is an exemplary case where teams achieved top results using exceptional data preprocessing and augmentation techniques, rather than the volume of data.
Iteratively validate and tune the model, not just after it’s completion. It’s advisable to test the model at multiple stages of its development. The well-documented success of Microsoft’s The Decision Forest algorithm, which won the prestigious GE Flight Quest 2 competition, largely stemmed from a systematic and iterative validation process.
Remember, these practices serve as a guideline, not a strict rulebook. Adapt and modify based on requirements, keeping in mind that the ultimate goal is to produce robust, efficient, and morally sound ML models.
The Future of Machine Learning
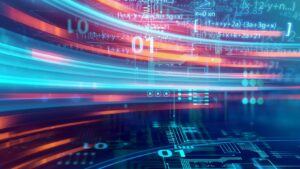
With its ever-evolving footprint, Machine Learning (ML) taps into an extensively expanding set of industries. The scope includes advanced fields such as Quantum Computing, challenging the limits of conventional ML models, and the Internet of Things (IoT), fueling a new age of smart appliances.
Machine Learning’s foray into Quantum Computing represents a pivotal shift. Quantum computers, like IBM’s Q System One, are designed to decipher complex problems unconquerable by classical computing. Tasked with processing large, high-dimensional datasets, these machines can potentially magnify ML capabilities.
In this dynamic unfolding of Machine Learning’s future, the potential shown is colossal. However, the roadmap requires addressing emerging challenges, ensuring ethical usage, and upholding user trust and understanding.