Predicting demand fluctuations is a critical aspect of effective logistics and supply chain management. Accurate demand forecasting enables companies to optimize inventory levels, reduce costs, improve customer satisfaction, and maintain a competitive edge. With the rise of advanced technologies and data analytics, organizations now have more tools at their disposal to predict and respond to changes in demand.
This article explores the methodologies, technologies, and strategies employed to forecast demand fluctuations in logistics and supply chains.
The Importance of Demand Forecasting
Demand forecasting in logistics and supply chains involves predicting future customer demand for products and services. Accurate demand forecasting is essential for several reasons:
- Inventory management: It helps maintain optimal inventory levels, avoiding both stockouts and overstock situations.
- Cost reduction: Efficient demand forecasting reduces warehousing and transportation costs.
- Customer satisfaction: Ensuring product availability leads to higher customer satisfaction and loyalty.
- Resource allocation: It allows better allocation of resources such as labor, machinery, and storage space.
- Strategic planning: Provides insights for long-term planning and decision-making.
Demand forecasting is crucial in addressing modern supply chain challenges, as it enables organizations to navigate complexities and uncertainties with greater precision and agility. In today’s fast-paced market environment, characterized by globalization, fluctuating consumer preferences, and unpredictable disruptions such as pandemics or geopolitical events, accurate demand forecasting helps companies maintain optimal inventory levels, reducing the risk of stockouts or excess stock.
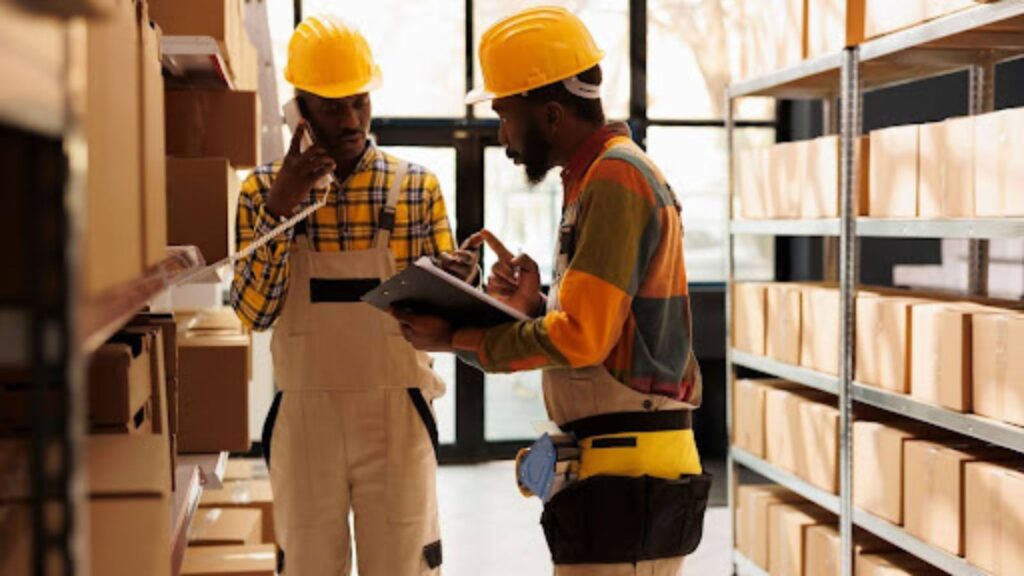
This, in turn, minimizes storage costs and maximizes cash flow. Moreover, effective demand forecasting enhances customer satisfaction by ensuring product availability and timely delivery, which is vital for maintaining competitive advantage. It also facilitates better planning and resource allocation, allowing companies to optimize production schedules, workforce deployment, and transportation logistics.
As supply chain becomes more interconnected and data-driven, integrating advanced technologies like AI and big data analytics into demand forecasting processes provides actionable insights, enabling businesses to anticipate and respond proactively to market changes and potential disruptions. Thus, demand forecasting is not just a predictive tool but a strategic enabler for resilient and efficient supply chain management.
Methods of Demand Forecasting
Several methods are used to predict demand fluctuations, ranging from traditional statistical models to advanced machine learning algorithms. These methods can be broadly classified into quantitative and qualitative approaches:
Quantitative Methods
Quantitative methods rely on numerical data and statistical techniques to predict future demand, leveraging historical sales data to identify patterns and relationships. These methods, including moving averages, regression analysis, and machine learning algorithms, provide objective and data-driven forecasts that can be highly accurate when sufficient data is available. Quantitative methods include the following practices:
- Time Series Analysis (TSA): This involves analyzing historical data to identify patterns or trends. Common techniques include moving averages, exponential smoothing, and ARIMA (Auto-Regressive Integrated Moving Average) models.
- Causal models: These models, such as regression analysis, examine the relationship between demand and other variables like price, economic indicators, and marketing efforts.
- Machine learning: Advanced algorithms, including neural networks, support vector machines, and ensemble methods, can handle large datasets and capture complex patterns to make accurate predictions.
Qualitative Methods
Such methods are based on subjective judgment and expert opinion. They utilize insights from market research, expert panels, and customer feedback to predict demand, often capturing nuances and contextual factors that quantitative models might overlook. While quantitative methods are precise and scalable, qualitative methods are flexible and valuable in scenarios where historical data is sparse or rapidly changing. Combining both approaches can enhance the robustness and reliability of demand forecasting. Qualitative methods involve such areas as:
- Expert Opinion: Every process related to gathering insights from industry experts, sales teams, and market analysts to forecast demand.
- Market Research: Surveys, focus groups, and customer feedback provide valuable information about future demand trends.
- Delphi Method: A structured communication technique where a panel of experts answers questionnaires in multiple rounds to reach a consensus on demand forecasts.
Leveraging Technology for Demand Forecasting
Technological advancements have revolutionized demand forecasting in logistics and supply chains. Key technologies include:
- Big data analytics: By analyzing large volumes of structured and unstructured data, companies can uncover hidden patterns and correlations that traditional methods might miss.
- Artificial Intelligence (AI) and Machine Learning: These technologies enhance predictive accuracy by learning from data and improving over time. They can process various data sources, such as social media trends, economic indicators, and weather patterns, to predict demand.
- Blockchain: Enhances transparency and traceability in the supply chain, ensuring data integrity and improving the reliability of demand forecasts.
- Cloud computing: Provides scalable computing resources and data storage, facilitating the processing and analysis of large datasets required for accurate demand forecasting.
Technology plays a transformative role in demand forecasting, enhancing accuracy and efficiency through advanced data analytics and computational power and equipping businesses with powerful tools to anticipate demand more accurately, respond swiftly to market changes, and maintain a resilient and efficient supply chain.
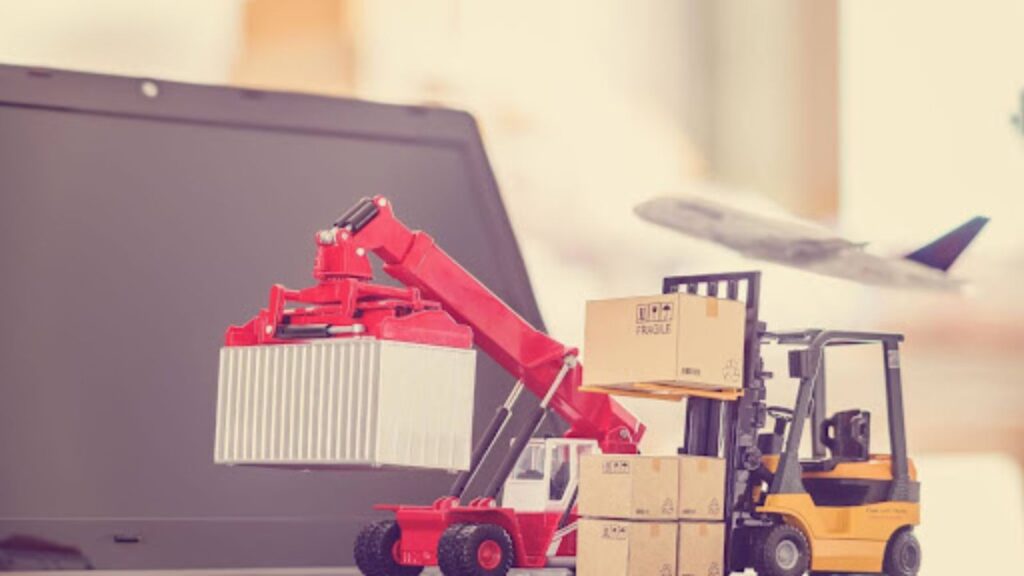
Strategies for Managing Demand Fluctuations
In addition to accurate forecasting, companies must develop strategies to manage demand fluctuations effectively:
- Agile supply chain: Developing a flexible supply chain that can quickly respond to changes in demand.
- Safety stock: Maintaining a buffer inventory to protect against unexpected demand spikes or supply chain disruptions.
- Collaborative planning: Working closely with suppliers and customers to align forecasts and share information for better demand visibility.
- Demand shaping: Influencing demand through pricing strategies, promotions, and marketing efforts to align with supply capabilities.
- Real-time monitoring: Using real-time data and analytics to continuously monitor and adjust to demand changes.
Challenges and Future Directions
Despite the advancements in technology and methodologies, demand forecasting in logistics and supply chains faces several challenges:
- Data quality: Ensuring the accuracy, completeness, and timeliness of data used for forecasting.
- Integration: Integrating data from various sources and systems across the supply chain.
- Uncertainty: Managing the inherent uncertainty and volatility in demand due to external factors like economic shifts, natural disasters, and geopolitical events.
- Skill gap: Addressing the shortage of skilled professionals who can interpret complex data and leverage advanced forecasting technologies.
Looking forward, the future of demand forecasting lies in further advancements in AI and machine learning, greater use of IoT and real-time data, and enhanced collaboration across the supply chain. Companies that invest in these technologies and strategies will be better equipped to predict and manage demand fluctuations, ensuring resilience and efficiency in their logistics and supply chain operations.
Conclusion
Predicting demand fluctuations is a complex but essential task in logistics and supply chains. By leveraging advanced technologies and adopting strategic approaches, companies can improve the accuracy of their demand forecasts, optimize their operations, and enhance their ability to respond to market changes. As the landscape continues to evolve, staying at the forefront of demand forecasting innovations and working with trusted technology partners for proper supply chain transformation will be crucial for maintaining a competitive advantage and achieving long-term success.