Retrieval-Augmented Generation (RAG) represents a significant leap forward in artificial intelligence technology. Unlike traditional AI models that rely solely on pre-trained data, RAG integrates a retrieval mechanism that accesses external knowledge bases in real-time. This process enhances the AI’s ability to provide more accurate and contextually relevant information. The importance of RAG in modern AI cannot be overstated, as it bridges the gap between static knowledge and dynamic, real-world data, making it a pivotal tool in intelligent information systems.To delve deeper into the specifics of this technology, explore what is RAG in AI and how it works.
How RAG Works
RAG works by combining a retrieval mechanism with a generation model, enabling the AI to fetch pertinent data from vast knowledge repositories. This integration allows for real-time information augmentation, ensuring that the responses generated are not only relevant but also up-to-date.
- Retrieval Mechanism Overview: The retrieval component is designed to search through large-scale databases and extract necessary information that aids the generation process.
- Integration of External Knowledge Bases: By linking to external knowledge bases, RAG can access a wealth of information beyond its initial training scope, allowing it to generate more informed responses.
- Real-Time Information Augmentation: This capability ensures that the AI’s output remains current and applicable to ongoing situations, significantly enhancing its utility in dynamic environments.
Core Components of RAG Systems
The efficacy of RAG systems is anchored on three core components:
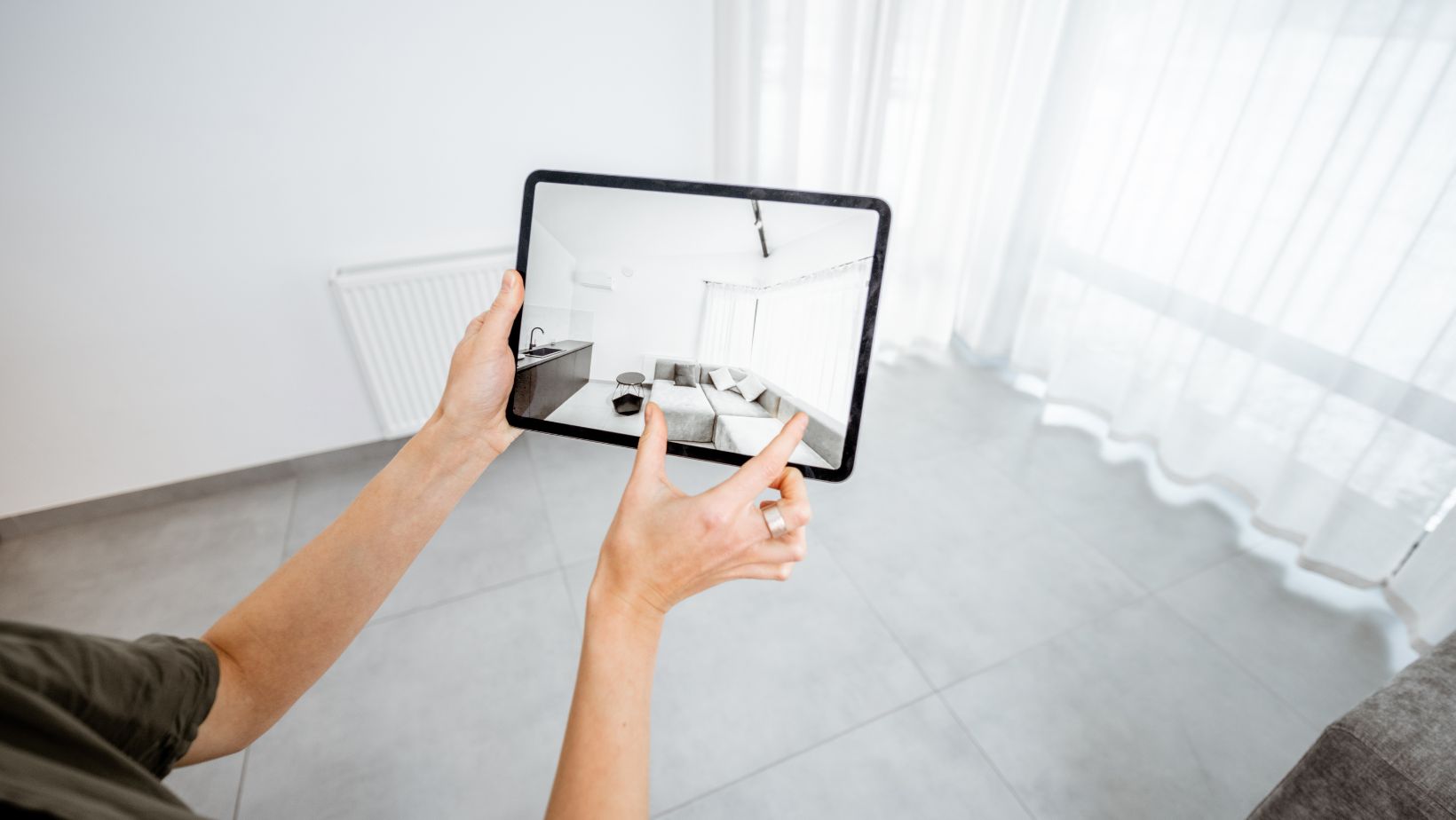
- Retrieval Mechanism: This component is responsible for searching and extracting relevant data from external sources.
- Generation Model: Once the data is retrieved, the generation model crafts coherent responses or outputs based on the information.
- Knowledge Integration Techniques: These techniques ensure seamless integration of retrieved data into the generation process, enhancing the quality and relevance of the AI’s output.
Industrial Applications of RAG
RAG technology’s versatility allows it to be employed across various industries, addressing complex information retrieval challenges and empowering decision-making processes.
Enterprise Knowledge Management
In the realm of enterprise knowledge management, RAG facilitates intelligent document retrieval and contextual information synthesis, making organizational knowledge more accessible and actionable.
- Intelligent Document Retrieval: RAG can efficiently navigate through large volumes of documents, pinpointing and retrieving the necessary data.
- Contextual Information Synthesis: By synthesizing information within context, RAG enhances the quality of insights derived from enterprise data.
- Improved Organizational Knowledge Access: This leads to streamlined access to critical information, boosting productivity and informed decision-making.
Customer Support and Service
RAG’s capabilities are particularly transformative in customer support and service, where personalized response generation and real-time information retrieval significantly reduce support response times.
- Personalized Response Generation: Tailoring responses to individual customer queries enhances user satisfaction and engagement.
- Real-Time Information Retrieval: This ensures that customer support agents have the most current data at their disposal, improving service quality.
- Reducing Support Response Times: By automating and optimizing the information retrieval process, RAG helps in delivering quicker solutions to customer issues. Learn more about improving customer service with AI here.
Technical Challenges and Future Developments
Despite its transformative potential, RAG technology faces several technical challenges that researchers and developers are actively working to overcome.
Performance Optimization Strategies
Current limitations in RAG systems revolve around improving retrieval accuracy, reducing computational overhead, and enhancing contextual understanding.
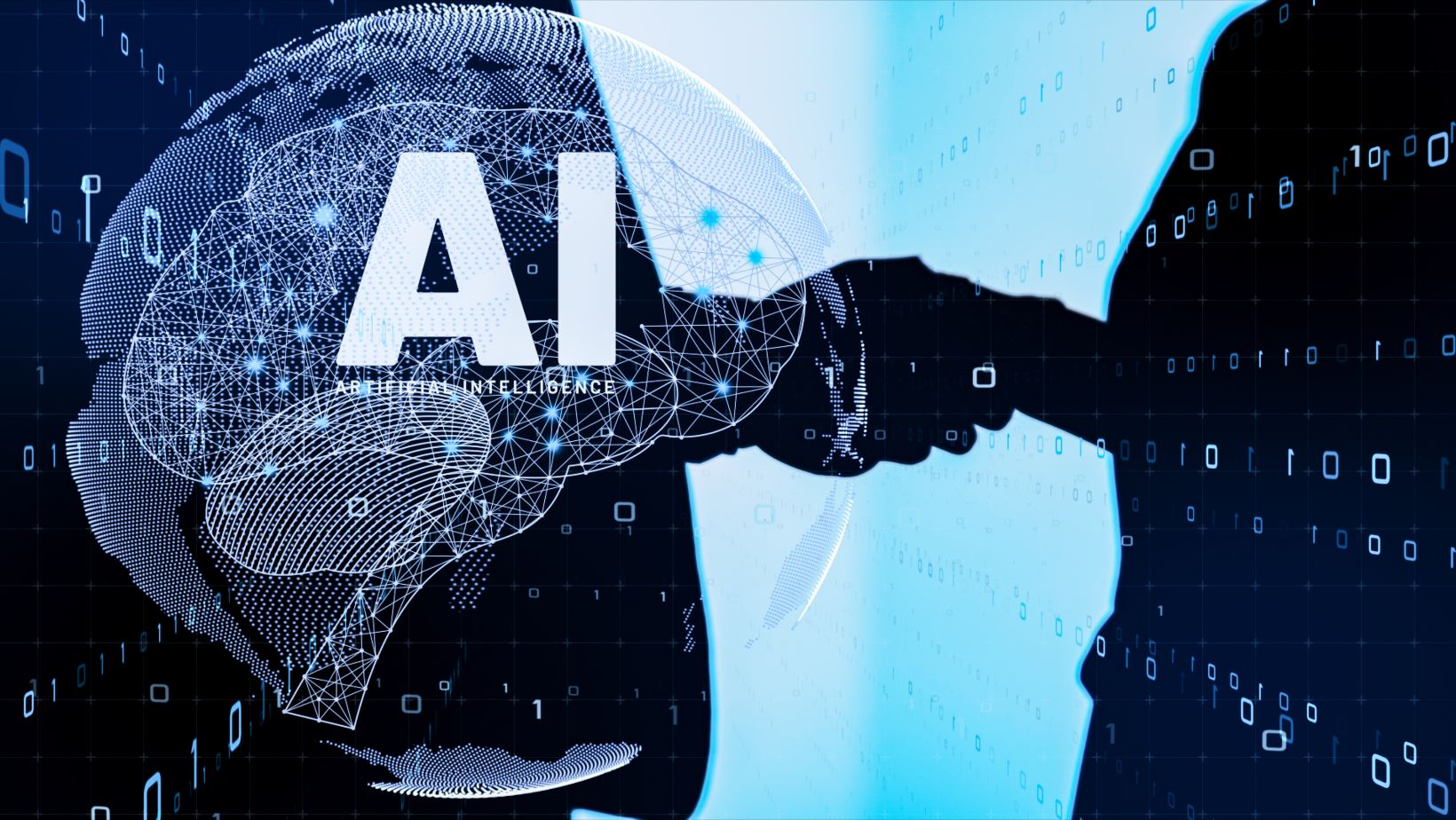
- Improving Retrieval Accuracy: Ensuring that the most relevant data is retrieved is crucial for the success of RAG systems.
- Reducing Computational Overhead: Streamlining the process to minimize resource consumption without sacrificing performance is a key challenge.
- Enhancing Contextual Understanding: Developing models that can interpret and utilize context more effectively is an ongoing area of research.
Emerging RAG Architectures
The future of RAG technology is promising, with exciting developments in multi-modal systems, domain-specific models, and advanced knowledge integration techniques.
- Multi-Modal RAG Systems: These systems integrate various types of data (e.g., text, images) to provide richer, more comprehensive outputs.
- Domain-Specific RAG Models: Tailoring RAG systems to specific industries or fields can enhance their effectiveness and precision.
- Advanced Knowledge Integration Techniques: Innovating how external data is integrated into the generation process can lead to significant improvements in output quality.
In conclusion, the advancement of RAG technology holds the potential to revolutionize various sectors by enhancing data retrieval and generation processes. As ongoing research addresses current limitations, the future of RAG appears bright, promising smarter, more efficient AI systems across industries.