Artificial intelligence has seeped into the mainstream and has become a super popular tech in the past two years. While for many, it may seem that this technology came out of nowhere. Yet, it has been in development for a while, with the first algorithms capable of logical operations getting created as early as the 1960s. However, the explosion of what we now call deep learning came in the 2010s, when the convolutional and recurrent neural networks found their applications in things like image/speech recognition and natural language processing. Now, using tools like MidJourney, Stable Diffusion, ChatGPT, Bard, and Grok is something virtually everyone does for daily work tasks or entertainment.
One area of AI that finds an ever-growing application is predictive machine learning, utilizing statistical analysis to identify patterns that help generate event forecasts. Algorithms can analyze vast data sets faster and more accurately than humans can, and they can spot commonalities between them that may not be as obvious. Hence, artificial intelligence can be far more efficient in accurately guessing future outcomes, especially given that AI models can learn from data over time and tweak their used parameters based on what they detect, which leads to improved accuracy over time. Below, we explore four fields where predictive AI has found implementation and staggering success rates.
Stock Predictions
The financial assets market is famous for its inherent complexity and volatility. It is not always clear what trends are active regarding stock price movements, making it difficult for traders and portfolio management services to know where to invest. Factors like politics, unplanned events, global economic conditions, investor sentiment, and more can dramatically affect how things will pan out in the short and long term.
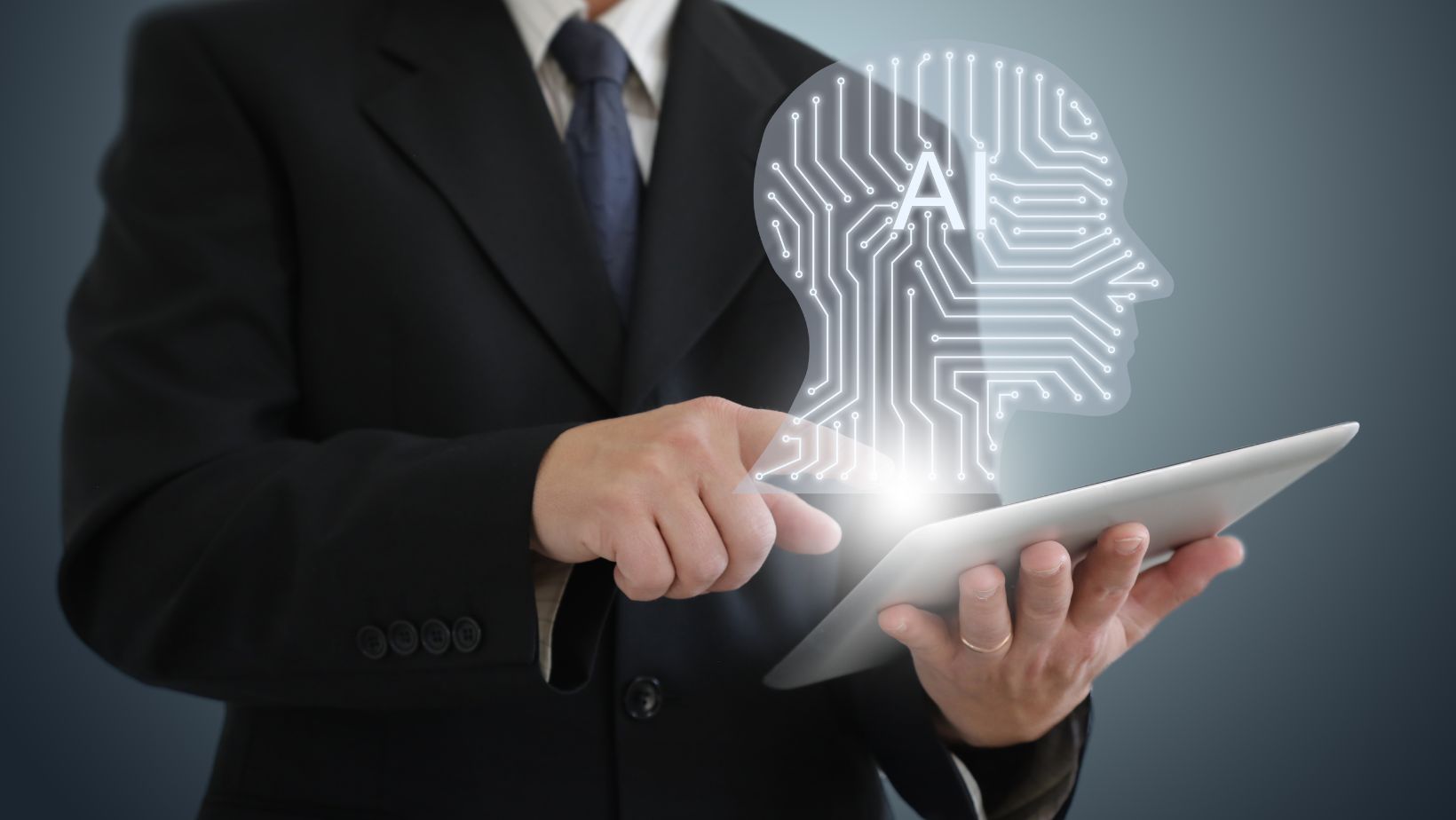
Enter AI. According to University Kaiserslautern-Landau professor Vitor Azevedo, high-end AI models can produce monthly stock returns of up to 2.71%, which is 1.71% higher than traditional methods. His findings, deduced from 1.9 billion stock-month anomalies analyzed for a twenty-nine-year period, were presented in a study titled Stock Market Anomalies and Machine Learning Across the Globe, published in the Journal of Asset Management.
Gambling Predictions
In recent times, AI apps for gambling predictions have become all the rage, with many online game-of-chance enthusiasts turning to Chat GPT for roulette picks and Gemini for their decisions regarding craps play. That said, gaming action is ultimately entirely chance-based, with results in online variations of casino classics’ generated through pseudo-random number generators whose behaviors can be impossible to foretell with any degree of certainty. Thus, most gamblers turn to implementing AI in sports outcome predictions, as these events can far more efficiently get prognosticated since they feature extensive historical datasets validly indicative of what may happen. Employing different methodologies can aid in extracting meaningful patterns from the information processed to come to a reasonably precise scenario of what may happen.
Various studies have been run on this topic, and different AI/machine learning models have been created for sports predictions. One of the more notable ones is Dolores, which guesses football match results using a combo of Hybrid Bayesian Networks and dynamic ratings, boasting a predictive error of 0.94%. Most sports betting models utilize Bayesian logistic regression, a statistical method that blends logistic regression with Bayesian inference. The latter combines prior beliefs with novel data to form updated guesses.
Weather Prediction
While some experts claim that artificial intelligence algorithms still cannot predict the weather as well as humans can, H. Jesse Smith, editor of Science Magazine and a University of California PhD, notes that GraphCast, an AI model developed by Google DeepMind, can foretell weather variables better than standard methods at high resolution, up to ten days in advance, proven in 90% of tested cases at 0.25° resolution.
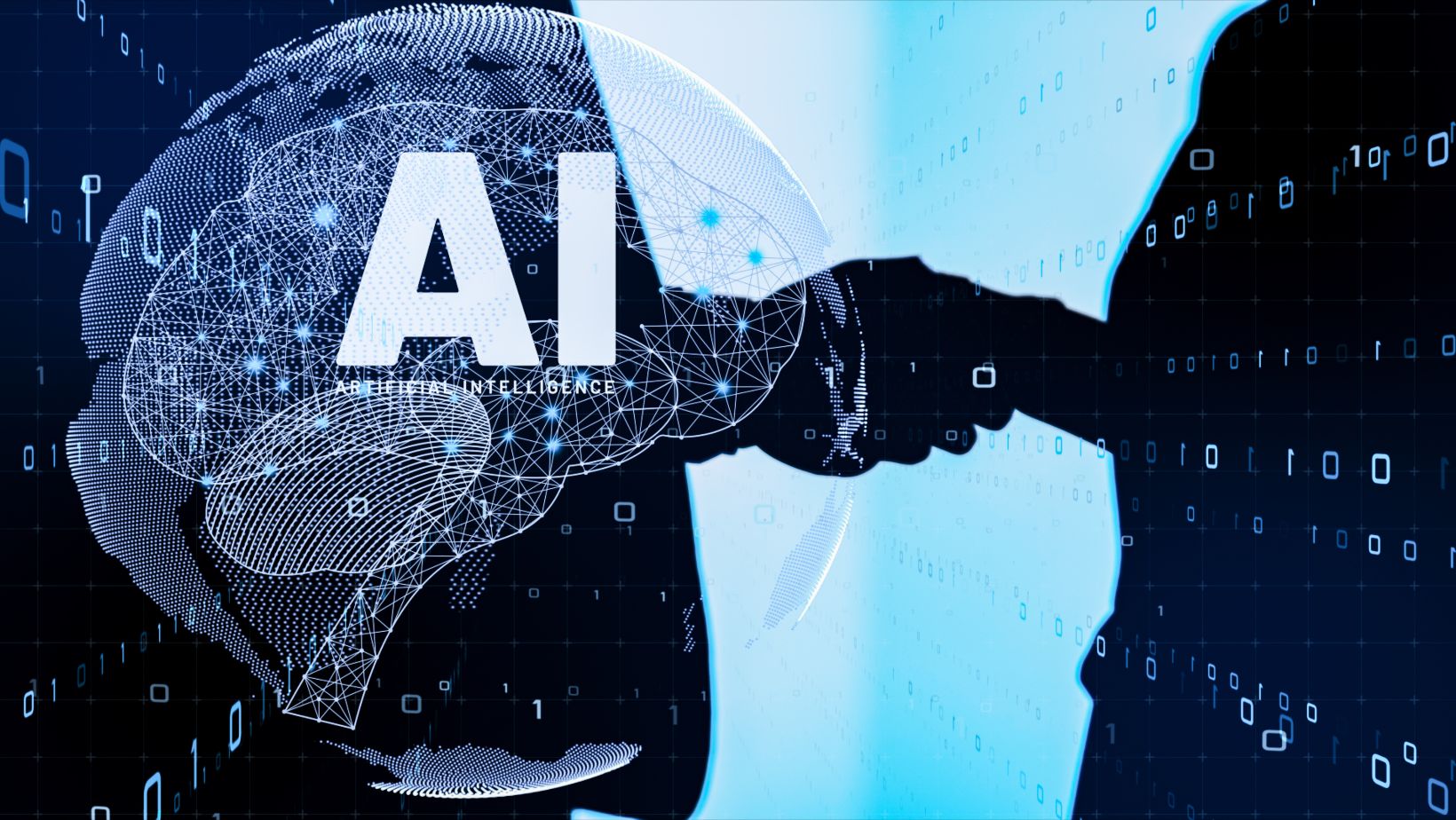
GraphCast utilizes an encoder-processor-decoder configuration, operating on a multi-mesh representation of the Earth’s surface. Per the current research, it is superior to established models like high-resolution ECMWF forecasting, achieving better Root Mean Square Error and Anomaly Correlation Coefficients. Nonetheless, University of Arizona professor Kim Wood believes that GraphCast’s overreliance on past data makes it poorly equipped to incorporate never-before-seen events and forecast rare happenings, making it inferior to human analysis, which is more intuitive and adaptive.
Content Consumption Predictions
The vast majority of content consumed on various platforms, including social media, comes from recommendation algorithms. These gather data from user interactions and run this information through collaborative filtering and deep learning to analyze and interpret it. Based on the constructed findings, matches consider factors like time of day, location, and device type. These pieces of software are invaluable for services and marketers, and like the previously discussed ones, they are continuously learning from user feedback to improve prediction accuracy.
Businesses now incorporate these recommendation systems to optimize user engagement and increase content consumption. Netflix claims that 80% of its viewed shows, movies, and documentaries get watched thanks to personalized recommendations made by its algorithms, which analyze user preferences. It is incredibly sophisticated and does not only look at things that meet a user’s taste but also looks to suggest trendy content. A recent McKinsey report states that customer satisfaction can get boosted by as much as 20% through effective personalization, which is why so many services are leaning towards it.